Stephen Beal
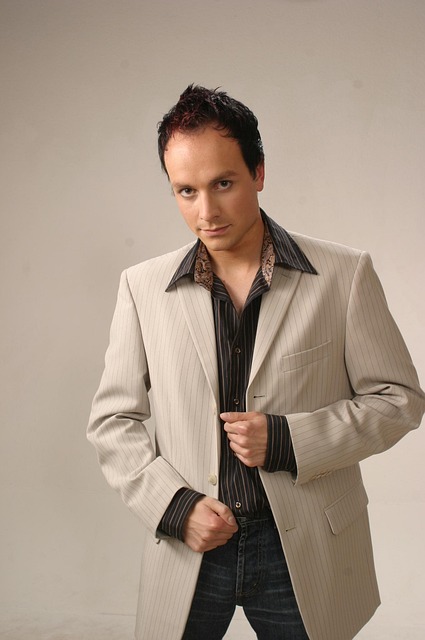
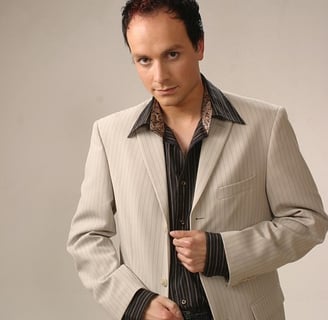
Name: Stephen Beal
Role: AI-Driven Smart Warehousing Specialist
Expertise: Optimizing Warehouse Layouts and Picking Processes with AI
Professional Summary:
Stephen Beal is an innovative professional in the field of AI-driven smart warehousing, specializing in leveraging artificial intelligence to optimize warehouse layouts and picking processes. With a strong foundation in logistics, data science, and machine learning, Stephen is dedicated to transforming traditional warehousing operations by integrating cutting-edge AI technologies. His work enhances efficiency, reduces operational costs, and improves accuracy in inventory management and order fulfillment, enabling businesses to achieve greater productivity and customer satisfaction.
Key Competencies:
AI-Powered Warehouse Layout Optimization:
Develops advanced AI algorithms to analyze spatial data, inventory flow, and operational needs, designing optimal warehouse layouts for maximum efficiency.
Utilizes machine learning to dynamically adjust layouts based on changing demand patterns and seasonal variations.
Intelligent Picking Process Automation:
Designs AI-driven systems to optimize picking routes, reduce travel time, and enhance order accuracy.
Implements robotic and AI-assisted picking solutions to streamline operations and reduce manual labor.
Data Integration & Analytics:
Proficient in integrating and analyzing data from multiple sources, including inventory systems, IoT sensors, and order histories, to provide actionable insights.
Builds scalable data pipelines to process and interpret large datasets for continuous improvement of warehousing processes.
Machine Learning Expertise:
Builds and optimizes machine learning models for warehousing applications, including demand forecasting, inventory classification, and route optimization.
Stays updated with the latest AI advancements to drive innovation in smart warehousing.
Cross-Functional Collaboration:
Collaborates with warehouse managers, logistics teams, and business leaders to align AI solutions with organizational goals.
Provides training and support to ensure the successful adoption of AI tools.
Career Highlights:
Developed an AI-powered warehouse layout optimization system that increased storage efficiency by 20% for a leading retail company.
Designed an intelligent picking process framework that reduced order fulfillment time by 15% for a global e-commerce platform.
Published influential research on AI applications in smart warehousing, earning recognition at international logistics conferences.
Personal Statement:
"I am passionate about leveraging AI to revolutionize warehousing operations, enabling businesses to operate more efficiently and responsively. My mission is to create data-driven solutions that optimize layouts and processes, driving cost savings and customer satisfaction."
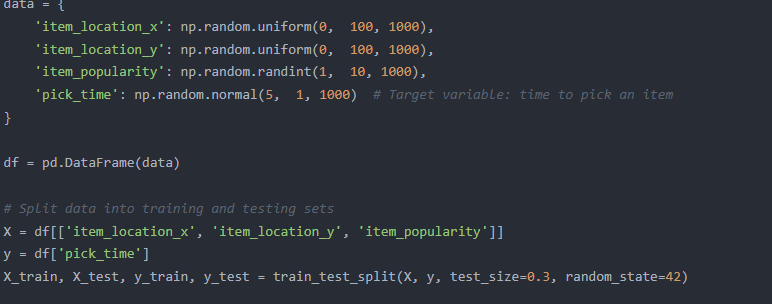
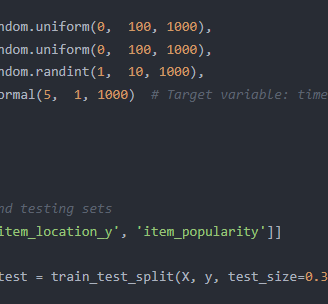
Fine-Tuning Necessity
Fine-tuning GPT-4 is essential for this research because publicly available GPT-3.5 lacks the specialized capabilities required for analyzing complex warehouse data and generating optimized layout and picking strategies. Intelligent warehousing involves highly domain-specific knowledge, intricate operational patterns, and nuanced optimization criteria that general-purpose models like GPT-3.5 cannot adequately address. Fine-tuning GPT-4 allows the model to learn from intelligent warehousing datasets, adapt to the unique challenges of the domain, and provide more accurate and actionable insights. This level of customization is critical for advancing AI’s role in warehousing optimization and ensuring its practical utility in real-world scenarios.
Past Research
To better understand the context of this submission, I recommend reviewing my previous work on the application of AI in warehousing optimization, particularly the study titled "Enhancing Warehouse Efficiency Using Machine Learning Models." This research explored the use of simulation-based optimization and reinforcement learning techniques for improving warehouse operations. Additionally, my paper "Adapting Large Language Models for Domain-Specific Applications in Intelligent Warehousing" provides insights into the fine-tuning process and its potential to enhance model performance in specialized fields.